Introduction to Pandas
Contents
Introduction to Pandas¶
Before we jump into Pandas, let us review what we have considered so far.
First, we learned how to read data from files into numpy arrays. We learned how to use variables to store that data, and to either slice the array into a few variables, or use slices themselves for something. We also learned how to make a record array that enabled us to access columns of the array by a name.
When we loaded a json file, we got a dictionary data structure, which also allowed us to access data by a name.
Second, we imported a visualization library, and made plots that used the arrays as arguments.
For “small” data sets, i.e. not too many columns, this is a perfectly reasonable thing to do. For larger datasets, however, it can be tedious to create a lot of variable names, and it is also hard to remember what is in each column.
Many tasks are pretty standard, e.g. read a data set, summarize and visualize it. It would be nice if we had a simple way to do this, with few lines of code, since those lines will be the same every time.
The Pandas library was developed to address all these issues. From the website: “pandas is a fast, powerful, flexible and easy to use open source data analysis and manipulation tool, built on top of the Python programming language.”
Review of the numpy array way¶
Let’s review what we learned already.
import matplotlib.pyplot as plt
import numpy as np
data = np.loadtxt("raman.txt")
wavenumber, intensity = data.T # the transpose has data in rows for unpacking
ind = (wavenumber >= 1000) & (wavenumber < 1500)
plt.figure()
plt.plot(wavenumber[ind], intensity[ind])
plt.xlabel("Wavenumber")
plt.ylabel("Intensity");
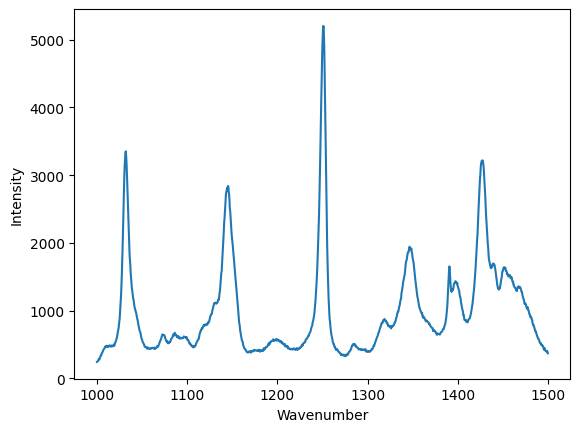
Now, with Pandas¶
We will unpack this code shortly. For now, look how short it is to create this plot. Note that we have condensed all the code in the example above basically into three lines of code. That is pretty remarkable, but should give you some pause. We now have to learn how to use such a dense syntax!
import pandas as pd
df = pd.read_csv(
"raman.txt", delimiter="\t", index_col=0, names=["wavenumber", "intensity"]
)
df[(df.index >= 1000) & (df.index < 1500)].plot();
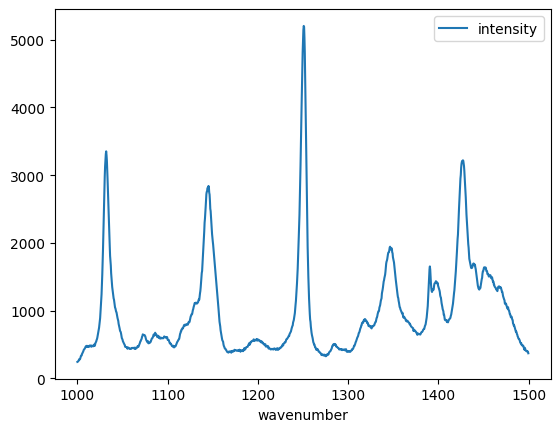
And to summarize.
df.describe()
intensity | |
---|---|
count | 7620.000000 |
mean | 558.388392 |
std | 982.659031 |
min | 77.104279 |
25% | 113.666535 |
50% | 219.409340 |
75% | 552.632848 |
max | 15275.059000 |
What is the benefit of this dense syntax? Because it is so short, it is faster to type (at least, when you know what to type). That means it is also faster for you to read.
The downside is that it is like learning a whole new language within Python, and a new mental model for how the data is stored and accessed. You have to decide if it is worthwhile doing that. If you do this a lot, it is probably worthwhile.
Pandas¶
The main object we will work with is called a DataFrame
.
type(df)
pandas.core.frame.DataFrame
Jupyter notebooks can show you a fancy rendering of your dataframe.
df
intensity | |
---|---|
wavenumber | |
500.00000 | 294.378690 |
500.39374 | 288.922000 |
500.78751 | 286.066220 |
501.18124 | 275.222840 |
501.57501 | 275.119380 |
... | ... |
3498.42500 | 86.151878 |
3498.81880 | 85.178947 |
3499.21240 | 87.969734 |
3499.60620 | 83.638931 |
3500.00000 | 84.009064 |
7620 rows Ă— 1 columns
The dataframe combines a few ideas we used from arrays and dictionaries. First, we can access a column by name. When we do this, we get a Series
object.
type(df["intensity"])
pandas.core.series.Series
You can extract the values into a numpy array like this.
df["intensity"].values
array([294.37869 , 288.922 , 286.06622 , ..., 87.969734, 83.638931,
84.009064])
A Series (and DataFrame) are like numpy arrays in some ways, and different in others. Suppose we want to see the first five entries of the intensity. If we want to use integer-based indexing like we have so far, you have to use the iloc
attribute on the series like this. iloc
is for integer location.
df["intensity"].iloc[0:5]
wavenumber
500.00000 294.37869
500.39374 288.92200
500.78751 286.06622
501.18124 275.22284
501.57501 275.11938
Name: intensity, dtype: float64
What about the wavenumbers? These are called the index of the dataframe.
df.index
Index([ 500.0, 500.39374, 500.78751, 501.18124, 501.57501, 501.96875,
502.36252, 502.75626, 503.15002, 503.54376,
...
3496.4563, 3496.8501, 3497.2437, 3497.6375, 3498.0313, 3498.425,
3498.8188, 3499.2124, 3499.6062, 3500.0],
dtype='float64', name='wavenumber', length=7620)
You can index the index with integers as you can with an array.
df.index[0:5]
Index([500.0, 500.39374, 500.78751, 501.18124, 501.57501], dtype='float64', name='wavenumber')
Finally, you can combine these so that you index a column with a slice of the index like this.
df["intensity"][df.index[0:5]]
wavenumber
500.00000 294.37869
500.39374 288.92200
500.78751 286.06622
501.18124 275.22284
501.57501 275.11938
Name: intensity, dtype: float64
In summary, we can think of a dataframe as a hybrid array/dictionary where we have an index which is like the independent variable, and a set of columns that are like dependent variables. You can access the columns like a dictionary.
Dataframes and visualization¶
Dataframes also provide easy access to visualization. The simplest method is to just call the plot method on a dataframe. Note this automatically makes the plot with labels and a legend. If there are many columns, you will have a curve for each one of them. We will see that later.
df.plot();
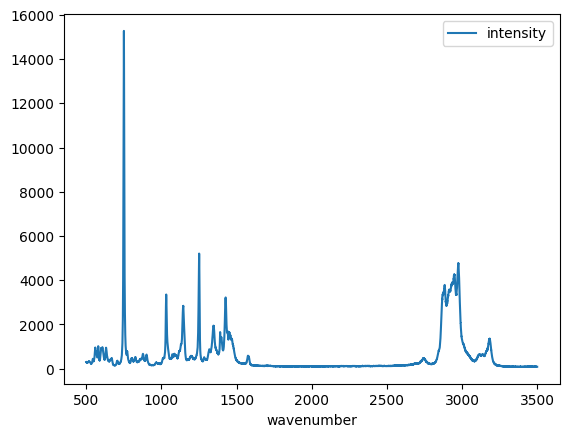
Reading data in Pandas¶
Let’s get back to how we got the data into Pandas. Let’s retrieve the data file we used before with several columns in it.
fname = "p-t.dat"
url = "https://www.itl.nist.gov/div898/handbook/datasets/MODEL-4_4_4.DAT"
import urllib.request
urllib.request.urlretrieve(url, fname);
Let’s refresh our memory of what is in this file:
! head p-t.dat
Run Ambient Fitted
Order Day Temperature Temperature Pressure Value Residual
1 1 23.820 54.749 225.066 222.920 2.146
2 1 24.120 23.323 100.331 99.411 0.920
3 1 23.434 58.775 230.863 238.744 -7.881
4 1 23.993 25.854 106.160 109.359 -3.199
5 1 23.375 68.297 277.502 276.165 1.336
6 1 23.233 37.481 148.314 155.056 -6.741
7 1 24.162 49.542 197.562 202.456 -4.895
8 1 23.667 34.101 138.537 141.770 -3.232
We use Pandas.read_csv to read this, similar to how we used numpy.loadtxt
. It also takes a lot of arguments to fine-tune the output. We use spaces as the delimiter here. '\s+'
is a regular expression for multiple spaces. We still skip two rows, and we have to manually define the column names. We do not specify an index column here, we get a default one based on integers. Pandas is smart enough to recognize the first two columns are integers, so we do not have to do anything special here.
df = pd.read_csv(
"p-t.dat",
delimiter="\s+",
skiprows=2,
names=[
"Run order",
"Day",
"Ambient Temperature",
"Temperature",
"Pressure",
"Fitted Value",
"Residual",
],
)
df
Run order | Day | Ambient Temperature | Temperature | Pressure | Fitted Value | Residual | |
---|---|---|---|---|---|---|---|
0 | 1 | 1 | 23.820 | 54.749 | 225.066 | 222.920 | 2.146 |
1 | 2 | 1 | 24.120 | 23.323 | 100.331 | 99.411 | 0.920 |
2 | 3 | 1 | 23.434 | 58.775 | 230.863 | 238.744 | -7.881 |
3 | 4 | 1 | 23.993 | 25.854 | 106.160 | 109.359 | -3.199 |
4 | 5 | 1 | 23.375 | 68.297 | 277.502 | 276.165 | 1.336 |
5 | 6 | 1 | 23.233 | 37.481 | 148.314 | 155.056 | -6.741 |
6 | 7 | 1 | 24.162 | 49.542 | 197.562 | 202.456 | -4.895 |
7 | 8 | 1 | 23.667 | 34.101 | 138.537 | 141.770 | -3.232 |
8 | 9 | 1 | 24.056 | 33.901 | 137.969 | 140.983 | -3.014 |
9 | 10 | 1 | 22.786 | 29.242 | 117.410 | 122.674 | -5.263 |
10 | 11 | 2 | 23.785 | 39.506 | 164.442 | 163.013 | 1.429 |
11 | 12 | 2 | 22.987 | 43.004 | 181.044 | 176.759 | 4.285 |
12 | 13 | 2 | 23.799 | 53.226 | 222.179 | 216.933 | 5.246 |
13 | 14 | 2 | 23.661 | 54.467 | 227.010 | 221.813 | 5.198 |
14 | 15 | 2 | 23.852 | 57.549 | 232.496 | 233.925 | -1.429 |
15 | 16 | 2 | 23.379 | 61.204 | 253.557 | 248.288 | 5.269 |
16 | 17 | 2 | 24.146 | 31.489 | 139.894 | 131.506 | 8.388 |
17 | 18 | 2 | 24.187 | 68.476 | 273.931 | 276.871 | -2.940 |
18 | 19 | 2 | 24.159 | 51.144 | 207.969 | 208.753 | -0.784 |
19 | 20 | 2 | 23.803 | 68.774 | 280.205 | 278.040 | 2.165 |
20 | 21 | 3 | 24.381 | 55.350 | 227.060 | 225.282 | 1.779 |
21 | 22 | 3 | 24.027 | 44.692 | 180.605 | 183.396 | -2.791 |
22 | 23 | 3 | 24.342 | 50.995 | 206.229 | 208.167 | -1.938 |
23 | 24 | 3 | 23.670 | 21.602 | 91.464 | 92.649 | -1.186 |
24 | 25 | 3 | 24.246 | 54.673 | 223.869 | 222.622 | 1.247 |
25 | 26 | 3 | 25.082 | 41.449 | 172.910 | 170.651 | 2.259 |
26 | 27 | 3 | 24.575 | 35.451 | 152.073 | 147.075 | 4.998 |
27 | 28 | 3 | 23.803 | 42.989 | 169.427 | 176.703 | -7.276 |
28 | 29 | 3 | 24.660 | 48.599 | 192.561 | 198.748 | -6.188 |
29 | 30 | 3 | 24.097 | 21.448 | 94.448 | 92.042 | 2.406 |
30 | 31 | 4 | 22.816 | 56.982 | 222.794 | 231.697 | -8.902 |
31 | 32 | 4 | 24.167 | 47.901 | 199.003 | 196.008 | 2.996 |
32 | 33 | 4 | 22.712 | 40.285 | 168.668 | 166.077 | 2.592 |
33 | 34 | 4 | 23.611 | 25.609 | 109.387 | 108.397 | 0.990 |
34 | 35 | 4 | 23.354 | 22.971 | 98.445 | 98.029 | 0.416 |
35 | 36 | 4 | 23.669 | 25.838 | 110.987 | 109.295 | 1.692 |
36 | 37 | 4 | 23.965 | 49.127 | 202.662 | 200.826 | 1.835 |
37 | 38 | 4 | 22.917 | 54.936 | 224.773 | 223.653 | 1.120 |
38 | 39 | 4 | 23.546 | 50.917 | 216.058 | 207.859 | 8.199 |
39 | 40 | 4 | 24.450 | 41.976 | 171.469 | 172.720 | -1.251 |
The default plot is not that nice.
df.plot();
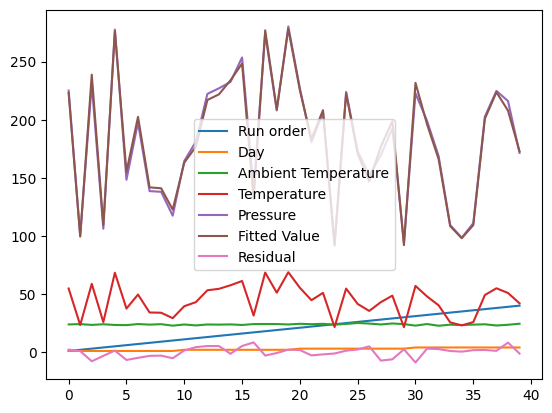
The default is to plot each column vs the index, which is not that helpful for us. Say we just want to plot the pressure vs. the temperature.
df.plot(x="Temperature", y="Pressure", style="b.");
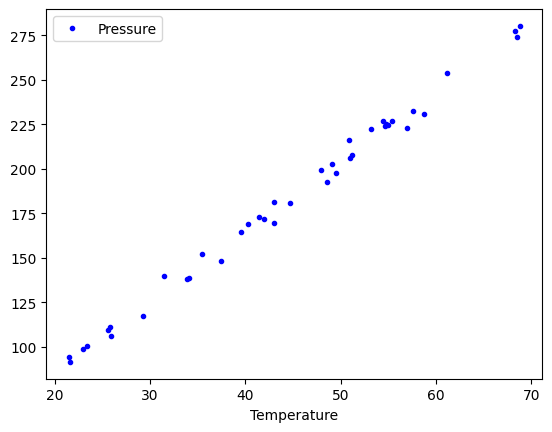
We can add multiple plots to a figure, but we have to tell the subsequent calls which axes to put them on. To do that, save the first one, and pass it as an argument in subsequent plots. That also allows you to fine-tune the plot appearance, e.g. add a y-label. See the matplotlib documentation to learn how to set all of these.
p1 = df.plot(x="Temperature", y="Pressure", style="b.")
df.plot(x="Temperature", y="Fitted Value", ax=p1)
p1.set_ylabel("values");
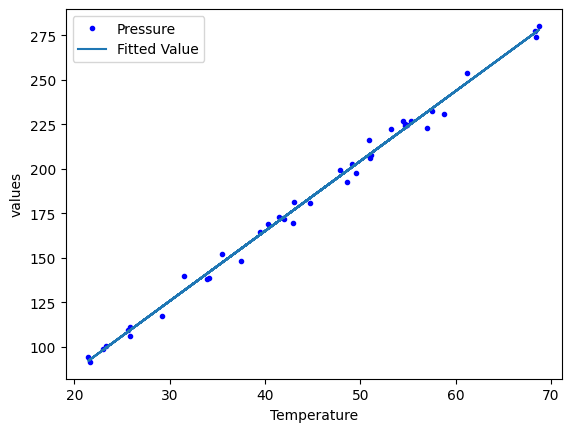
It is a reasonable question to ask if this is simpler than what we did before using arrays, variables and plotting commands. Dataframes are increasingly common in data science, and are the data structure used in many data science/machine learning projects.
Another real-life example¶
LAMMPS is a molecular simulation code used to run molecular dynamics. It outputs a text file that is somewhat challenging to read. There are variable numbers of time steps that depend on how the simulation was setup.
Start by downloading and opening this file. It is a molecular dynamics trajectory at constant volume, where the pressure, temperature and energy fluctuate.
Open this file log1.lammpsto get a sense for what is in it. The data starts around:
timestep 0.005
run ${runSteps}
run 500000
Per MPI rank memory allocation (min/avg/max) = 4.427 | 4.427 | 4.427 Mbytes
Step v_mytime Temp Press Volume PotEng TotEng v_pxy v_pxz v_pyz v_v11 v_v22 v_v33 CPU
0 0 1025 601.28429 8894.6478 -1566.6216 -1500.5083 2065.6285 1713.4095 203.00499 1.3408976e-05 9.2260011e-06 1.2951038e-07 0 w
And it ends around this line.
500000 2500 978.62359 -2100.7614 8894.6478 -1570.5382 -1507.4162 -252.80665 614.87398 939.65393 0.00045263648 0.00043970796 0.00044228719 1288.0233
Loop time of 1288.02 on 1 procs for 500000 steps with 500 atoms
Our job is to figure out where those lines are so we can read them into Pandas. There are many ways to do this, but we will stick with a pure Python way. The strategy is to search for the lines, and keep track of their positions.
start, stop = None, None
with open("log1.lammps") as f:
for i, line in enumerate(f):
if line.startswith("Step v_mytime"):
start = i
if line.startswith("Loop time of "):
stop = i - 1 # stop on the previous line
break
start, stop
(69, 2570)
This gets tricky. We want to skip the rows up to the starting line. At that point, the line numbers restart as far as Pandas is concerned, so the header is in line 0 then, and the number of rows to read is defined by the stop line minus the start line. The values are separated by multiple spaces, so we use a pattern to indicate multiple spaces. Finally, we prevent the first column from being the index column by setting index_col to be False. See https://pandas.pydata.org/pandas-docs/stable/reference/api/pandas.read_csv.html>for all the details.
df = pd.read_csv(
"log1.lammps",
skiprows=start,
header=0,
nrows=stop - start,
delimiter="\s+",
index_col=False,
)
df
/tmp/ipykernel_2195/86227813.py:1: ParserWarning: Length of header or names does not match length of data. This leads to a loss of data with index_col=False.
df = pd.read_csv(
Step | v_mytime | Temp | Press | Volume | PotEng | TotEng | v_pxy | v_pxz | v_pyz | v_v11 | v_v22 | v_v33 | CPU | |
---|---|---|---|---|---|---|---|---|---|---|---|---|---|---|
0 | 0 | 0 | 1025.00000 | 601.28429 | 8894.6478 | -1566.6216 | -1500.5083 | 2065.62850 | 1713.409500 | 203.00499 | 0.000013 | 0.000009 | 1.295104e-07 | 0.000000 |
1 | 200 | 1 | 1045.85100 | -1974.43580 | 8894.6478 | -1569.6934 | -1502.2352 | 2530.16720 | -2203.376800 | -2193.88770 | 0.000423 | -0.000564 | 9.109558e-04 | 0.505391 |
2 | 400 | 2 | 1050.44480 | 2974.54030 | 8894.6478 | -1564.3755 | -1496.6210 | 1446.73930 | 637.829780 | 1794.70610 | 0.001115 | 0.000125 | 4.583668e-04 | 1.018666 |
3 | 600 | 3 | 1071.37780 | 2386.37510 | 8894.6478 | -1566.4325 | -1497.3278 | 599.73943 | -462.748090 | 558.54192 | 0.000766 | 0.000387 | 3.082071e-04 | 1.532061 |
4 | 800 | 4 | 1055.52810 | -661.78795 | 8894.6478 | -1569.3172 | -1501.2348 | 1775.43870 | -1551.263500 | -493.01032 | 0.000569 | 0.000380 | 2.913082e-04 | 2.051839 |
... | ... | ... | ... | ... | ... | ... | ... | ... | ... | ... | ... | ... | ... | ... |
2496 | 499200 | 2496 | 977.95894 | -1747.91220 | 8894.6478 | -1570.0162 | -1506.9370 | 1411.85600 | 1154.883400 | -2265.50500 | 0.000452 | 0.000440 | 4.424962e-04 | 1285.960300 |
2497 | 499400 | 2497 | 1066.50870 | -77.15260 | 8894.6478 | -1568.3816 | -1499.5910 | 4071.88400 | 3847.295900 | -1279.02860 | 0.000452 | 0.000440 | 4.428113e-04 | 1286.474100 |
2498 | 499600 | 2498 | 1052.18860 | 1958.97410 | 8894.6478 | -1565.8013 | -1497.9343 | -2152.54460 | 925.775780 | -1162.76120 | 0.000453 | 0.000439 | 4.427215e-04 | 1286.987000 |
2499 | 499800 | 2499 | 1057.30140 | -1709.95670 | 8894.6478 | -1570.6800 | -1502.4832 | -1530.34090 | -71.479217 | 731.44735 | 0.000453 | 0.000440 | 4.423706e-04 | 1287.503200 |
2500 | 500000 | 2500 | 978.62359 | -2100.76140 | 8894.6478 | -1570.5382 | -1507.4162 | -252.80665 | 614.873980 | 939.65393 | 0.000453 | 0.000440 | 4.422872e-04 | 1288.023300 |
2501 rows Ă— 14 columns
Visualizing the data¶
Plot a column¶
The effort was worth it though; look how easy it is to plot the data!
df.plot(x="Step", y="Press");
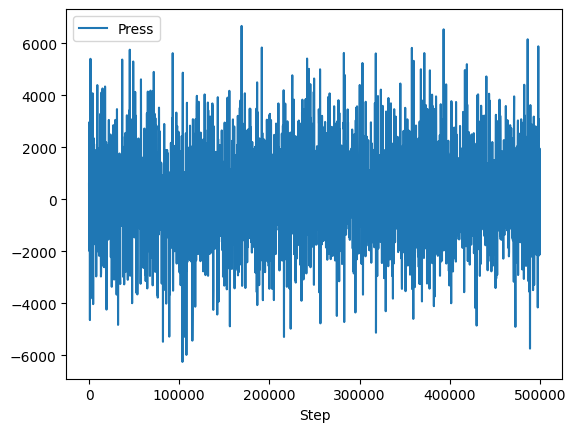
import matplotlib.pyplot as plt
fig, (ax0, ax1) = plt.subplots(1, 2)
df.plot(x="Temp", y="PotEng", style="b.", ax=ax0)
df.plot(x="Press", y="PotEng", style="b.", ax=ax1)
plt.tight_layout();
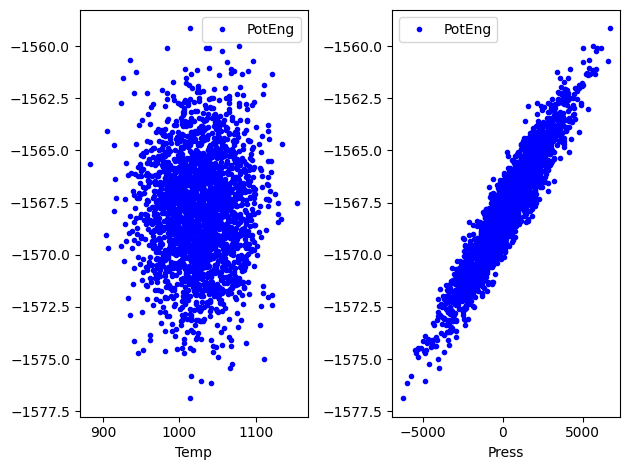
Plot distributions of a column¶
We can look at histograms of properties as easily.
df.hist("PotEng", xrot=45, bins=20, density=True);
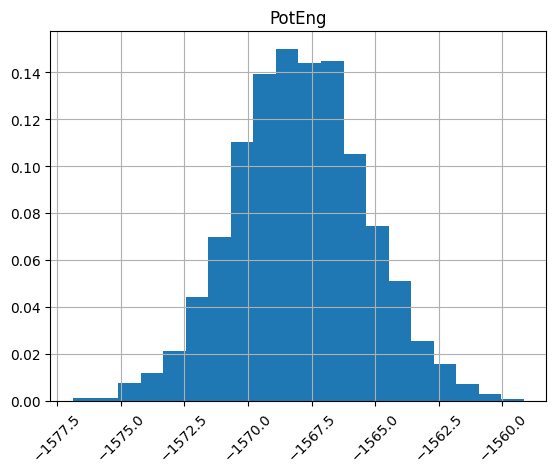
Plot column correlations¶
This is just the beginning of using Pandas. Suppose we want to see which columns are correlated (https://pandas.pydata.org/pandas-docs/stable/reference/api/pandas.DataFrame.corr.html). With variables this would be tedious.
plt.matshow(df.corr());
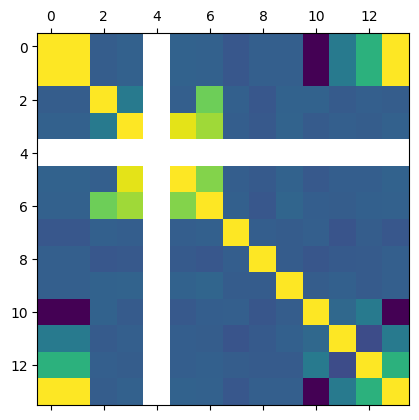
It can be helpful to see what these correlations mean. Here we plot all the columns against each other. Note, it is not possible to plot a column against itself with Pandas (I think this is a bug https://github.com/pandas-dev/pandas/issues/22088), so here I use matplotlib functions for the plotting. This should be symmetric, so I only plot the upper triangle.
keys = df.keys()
fig, axs = plt.subplots(13, 13)
fig.set_size_inches((8, 8))
for i in range(13):
for j in range(i, 13):
axs[i, j].plot(df[keys[i]], df[keys[j]], "b.", ms=2)
# remove axes so it is easier to read
axs[i, j].axes.get_xaxis().set_visible(False)
axs[i, j].axes.get_yaxis().set_visible(False)
axs[j, i].axes.get_xaxis().set_visible(False)
axs[j, i].axes.get_yaxis().set_visible(False)
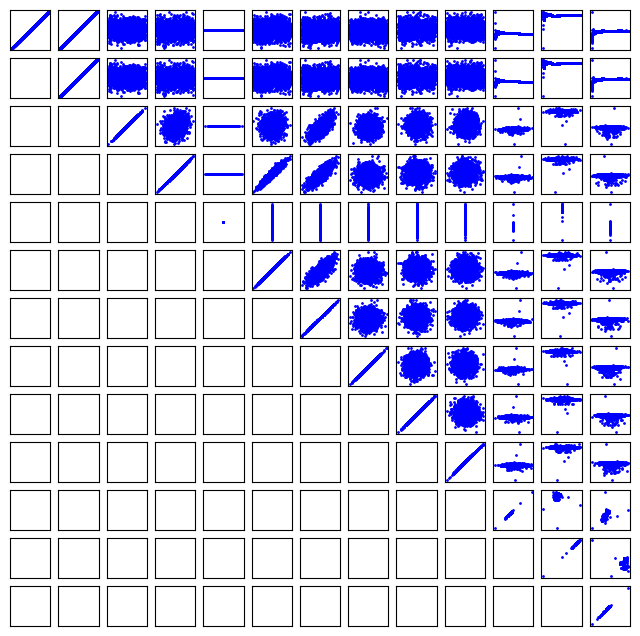
Getting parts of a Pandas DataFrame¶
We have seen how to get a column from a DataFrame like this:
df["Press"]
0 601.28429
1 -1974.43580
2 2974.54030
3 2386.37510
4 -661.78795
...
2496 -1747.91220
2497 -77.15260
2498 1958.97410
2499 -1709.95670
2500 -2100.76140
Name: Press, Length: 2501, dtype: float64
In this context, the DataFrame is acting like a dictionary. You can get a few columns by using a list of column names.
df[["Press", "PotEng"]]
Press | PotEng | |
---|---|---|
0 | 601.28429 | -1566.6216 |
1 | -1974.43580 | -1569.6934 |
2 | 2974.54030 | -1564.3755 |
3 | 2386.37510 | -1566.4325 |
4 | -661.78795 | -1569.3172 |
... | ... | ... |
2496 | -1747.91220 | -1570.0162 |
2497 | -77.15260 | -1568.3816 |
2498 | 1958.97410 | -1565.8013 |
2499 | -1709.95670 | -1570.6800 |
2500 | -2100.76140 | -1570.5382 |
2501 rows Ă— 2 columns
What about a row? This is what we would have done with a numpy array, but it just doesn’t work here.
Error
You get a KeyError here.
df[0]
---------------------------------------------------------------------------
KeyError Traceback (most recent call last)
File /opt/hostedtoolcache/Python/3.8.18/x64/lib/python3.8/site-packages/pandas/core/indexes/base.py:3653, in Index.get_loc(self, key)
3652 try:
-> 3653 return self._engine.get_loc(casted_key)
3654 except KeyError as err:
File /opt/hostedtoolcache/Python/3.8.18/x64/lib/python3.8/site-packages/pandas/_libs/index.pyx:147, in pandas._libs.index.IndexEngine.get_loc()
File /opt/hostedtoolcache/Python/3.8.18/x64/lib/python3.8/site-packages/pandas/_libs/index.pyx:176, in pandas._libs.index.IndexEngine.get_loc()
File pandas/_libs/hashtable_class_helper.pxi:7080, in pandas._libs.hashtable.PyObjectHashTable.get_item()
File pandas/_libs/hashtable_class_helper.pxi:7088, in pandas._libs.hashtable.PyObjectHashTable.get_item()
KeyError: 0
The above exception was the direct cause of the following exception:
KeyError Traceback (most recent call last)
Cell In[28], line 1
----> 1 df[0]
File /opt/hostedtoolcache/Python/3.8.18/x64/lib/python3.8/site-packages/pandas/core/frame.py:3761, in DataFrame.__getitem__(self, key)
3759 if self.columns.nlevels > 1:
3760 return self._getitem_multilevel(key)
-> 3761 indexer = self.columns.get_loc(key)
3762 if is_integer(indexer):
3763 indexer = [indexer]
File /opt/hostedtoolcache/Python/3.8.18/x64/lib/python3.8/site-packages/pandas/core/indexes/base.py:3655, in Index.get_loc(self, key)
3653 return self._engine.get_loc(casted_key)
3654 except KeyError as err:
-> 3655 raise KeyError(key) from err
3656 except TypeError:
3657 # If we have a listlike key, _check_indexing_error will raise
3658 # InvalidIndexError. Otherwise we fall through and re-raise
3659 # the TypeError.
3660 self._check_indexing_error(key)
KeyError: 0
The problem is that as a dictionary, the keys are for the columns.
df.keys()
Index(['Step', 'v_mytime', 'Temp', 'Press', 'Volume', 'PotEng', 'TotEng',
'v_pxy', 'v_pxz', 'v_pyz', 'v_v11', 'v_v22', 'v_v33', 'CPU'],
dtype='object')
One way to get the rows by their integer index is to use the integer location attribute for a row.
df.iloc[0]
Step 0.000000e+00
v_mytime 0.000000e+00
Temp 1.025000e+03
Press 6.012843e+02
Volume 8.894648e+03
PotEng -1.566622e+03
TotEng -1.500508e+03
v_pxy 2.065628e+03
v_pxz 1.713409e+03
v_pyz 2.030050e+02
v_v11 1.340898e-05
v_v22 9.226001e-06
v_v33 1.295104e-07
CPU 0.000000e+00
Name: 0, dtype: float64
We can use slices on this.
df.iloc[0:5]
Step | v_mytime | Temp | Press | Volume | PotEng | TotEng | v_pxy | v_pxz | v_pyz | v_v11 | v_v22 | v_v33 | CPU | |
---|---|---|---|---|---|---|---|---|---|---|---|---|---|---|
0 | 0 | 0 | 1025.0000 | 601.28429 | 8894.6478 | -1566.6216 | -1500.5083 | 2065.62850 | 1713.40950 | 203.00499 | 0.000013 | 0.000009 | 1.295104e-07 | 0.000000 |
1 | 200 | 1 | 1045.8510 | -1974.43580 | 8894.6478 | -1569.6934 | -1502.2352 | 2530.16720 | -2203.37680 | -2193.88770 | 0.000423 | -0.000564 | 9.109558e-04 | 0.505391 |
2 | 400 | 2 | 1050.4448 | 2974.54030 | 8894.6478 | -1564.3755 | -1496.6210 | 1446.73930 | 637.82978 | 1794.70610 | 0.001115 | 0.000125 | 4.583668e-04 | 1.018666 |
3 | 600 | 3 | 1071.3778 | 2386.37510 | 8894.6478 | -1566.4325 | -1497.3278 | 599.73943 | -462.74809 | 558.54192 | 0.000766 | 0.000387 | 3.082071e-04 | 1.532061 |
4 | 800 | 4 | 1055.5281 | -661.78795 | 8894.6478 | -1569.3172 | -1501.2348 | 1775.43870 | -1551.26350 | -493.01032 | 0.000569 | 0.000380 | 2.913082e-04 | 2.051839 |
This example may be a little confusing, because our index does include 0, so we can in this case also use the row label with the location attribute. You can use any value in the index for this.
df.index
RangeIndex(start=0, stop=2501, step=1)
df.loc[0]
Step 0.000000e+00
v_mytime 0.000000e+00
Temp 1.025000e+03
Press 6.012843e+02
Volume 8.894648e+03
PotEng -1.566622e+03
TotEng -1.500508e+03
v_pxy 2.065628e+03
v_pxz 1.713409e+03
v_pyz 2.030050e+02
v_v11 1.340898e-05
v_v22 9.226001e-06
v_v33 1.295104e-07
CPU 0.000000e+00
Name: 0, dtype: float64
We can access the first five rows like this.
df.loc[0:4]
Step | v_mytime | Temp | Press | Volume | PotEng | TotEng | v_pxy | v_pxz | v_pyz | v_v11 | v_v22 | v_v33 | CPU | |
---|---|---|---|---|---|---|---|---|---|---|---|---|---|---|
0 | 0 | 0 | 1025.0000 | 601.28429 | 8894.6478 | -1566.6216 | -1500.5083 | 2065.62850 | 1713.40950 | 203.00499 | 0.000013 | 0.000009 | 1.295104e-07 | 0.000000 |
1 | 200 | 1 | 1045.8510 | -1974.43580 | 8894.6478 | -1569.6934 | -1502.2352 | 2530.16720 | -2203.37680 | -2193.88770 | 0.000423 | -0.000564 | 9.109558e-04 | 0.505391 |
2 | 400 | 2 | 1050.4448 | 2974.54030 | 8894.6478 | -1564.3755 | -1496.6210 | 1446.73930 | 637.82978 | 1794.70610 | 0.001115 | 0.000125 | 4.583668e-04 | 1.018666 |
3 | 600 | 3 | 1071.3778 | 2386.37510 | 8894.6478 | -1566.4325 | -1497.3278 | 599.73943 | -462.74809 | 558.54192 | 0.000766 | 0.000387 | 3.082071e-04 | 1.532061 |
4 | 800 | 4 | 1055.5281 | -661.78795 | 8894.6478 | -1569.3172 | -1501.2348 | 1775.43870 | -1551.26350 | -493.01032 | 0.000569 | 0.000380 | 2.913082e-04 | 2.051839 |
And a slice of a column like this.
df.loc[0:4, "Press"]
0 601.28429
1 -1974.43580
2 2974.54030
3 2386.37510
4 -661.78795
Name: Press, dtype: float64
We can access a value in a row and column with the at
function on a DataFrame.
df.at[2, "Press"]
2974.5403
Or if you know the row and column numbers you can use iat
.
df.iat[2, 3]
2974.5403
Operating on columns in the DataFrame¶
Some functions just work across the columns. For example, DataFrames have statistics functions like this.
df.mean()
Step 250000.000000
v_mytime 1250.000000
Temp 1025.136938
Press 188.035304
Volume 8894.647800
PotEng -1567.935373
TotEng -1501.813207
v_pxy 16.669575
v_pxz -4.852837
v_pyz 14.175372
v_v11 0.000465
v_v22 0.000429
v_v33 0.000428
CPU 644.103768
dtype: float64
We should tread carefully with other functions that work on arrays. For example consider this example that computes the mean of an entire array.
a = np.array([[1, 1, 1], [2, 2, 2]])
np.mean(a)
1.5
It does not do the same thing on a DataFrame. The index and column labels are preserved with numpy functions.
import numpy as np
np.mean(df) # takes mean along axis 0
18497.01204734716
np.max(df)
500000.0
np.exp(df)
/opt/hostedtoolcache/Python/3.8.18/x64/lib/python3.8/site-packages/pandas/core/internals/blocks.py:329: RuntimeWarning: overflow encountered in exp
result = func(self.values, **kwargs)
Step | v_mytime | Temp | Press | Volume | PotEng | TotEng | v_pxy | v_pxz | v_pyz | v_v11 | v_v22 | v_v33 | CPU | |
---|---|---|---|---|---|---|---|---|---|---|---|---|---|---|
0 | 1.000000e+00 | 1.000000 | inf | 1.362854e+261 | inf | 0.0 | 0.0 | inf | inf | 1.458636e+88 | 1.000013 | 1.000009 | 1.000000 | 1.000000 |
1 | 7.225974e+86 | 2.718282 | inf | 0.000000e+00 | inf | 0.0 | 0.0 | inf | 0.000000e+00 | 0.000000e+00 | 1.000423 | 0.999436 | 1.000911 | 1.657634 |
2 | 5.221470e+173 | 7.389056 | inf | inf | inf | 0.0 | 0.0 | inf | 1.013804e+277 | inf | 1.001116 | 1.000125 | 1.000458 | 2.769498 |
3 | 3.773020e+260 | 20.085537 | inf | inf | inf | 0.0 | 0.0 | 2.907536e+260 | 1.074133e-201 | 3.729699e+242 | 1.000766 | 1.000387 | 1.000308 | 4.627705 |
4 | inf | 54.598150 | inf | 3.882801e-288 | inf | 0.0 | 0.0 | inf | 0.000000e+00 | 7.732831e-215 | 1.000569 | 1.000380 | 1.000291 | 7.782200 |
... | ... | ... | ... | ... | ... | ... | ... | ... | ... | ... | ... | ... | ... | ... |
2496 | inf | inf | inf | 0.000000e+00 | inf | 0.0 | 0.0 | inf | inf | 0.000000e+00 | 1.000452 | 1.000440 | 1.000443 | inf |
2497 | inf | inf | inf | 3.112086e-34 | inf | 0.0 | 0.0 | inf | inf | 0.000000e+00 | 1.000452 | 1.000440 | 1.000443 | inf |
2498 | inf | inf | inf | inf | inf | 0.0 | 0.0 | 0.000000e+00 | inf | 0.000000e+00 | 1.000453 | 1.000439 | 1.000443 | inf |
2499 | inf | inf | inf | 0.000000e+00 | inf | 0.0 | 0.0 | 0.000000e+00 | 9.056711e-32 | inf | 1.000453 | 1.000440 | 1.000442 | inf |
2500 | inf | inf | inf | 0.000000e+00 | inf | 0.0 | 0.0 | 1.612378e-110 | 1.087368e+267 | inf | 1.000453 | 1.000440 | 1.000442 | inf |
2501 rows Ă— 14 columns
2 * df
Step | v_mytime | Temp | Press | Volume | PotEng | TotEng | v_pxy | v_pxz | v_pyz | v_v11 | v_v22 | v_v33 | CPU | |
---|---|---|---|---|---|---|---|---|---|---|---|---|---|---|
0 | 0 | 0 | 2050.00000 | 1202.56858 | 17789.2956 | -3133.2432 | -3001.0166 | 4131.25700 | 3426.819000 | 406.00998 | 0.000027 | 0.000018 | 2.590208e-07 | 0.000000 |
1 | 400 | 2 | 2091.70200 | -3948.87160 | 17789.2956 | -3139.3868 | -3004.4704 | 5060.33440 | -4406.753600 | -4387.77540 | 0.000846 | -0.001128 | 1.821912e-03 | 1.010782 |
2 | 800 | 4 | 2100.88960 | 5949.08060 | 17789.2956 | -3128.7510 | -2993.2420 | 2893.47860 | 1275.659560 | 3589.41220 | 0.002231 | 0.000249 | 9.167335e-04 | 2.037332 |
3 | 1200 | 6 | 2142.75560 | 4772.75020 | 17789.2956 | -3132.8650 | -2994.6556 | 1199.47886 | -925.496180 | 1117.08384 | 0.001531 | 0.000774 | 6.164143e-04 | 3.064122 |
4 | 1600 | 8 | 2111.05620 | -1323.57590 | 17789.2956 | -3138.6344 | -3002.4696 | 3550.87740 | -3102.527000 | -986.02064 | 0.001137 | 0.000760 | 5.826165e-04 | 4.103678 |
... | ... | ... | ... | ... | ... | ... | ... | ... | ... | ... | ... | ... | ... | ... |
2496 | 998400 | 4992 | 1955.91788 | -3495.82440 | 17789.2956 | -3140.0324 | -3013.8740 | 2823.71200 | 2309.766800 | -4531.01000 | 0.000905 | 0.000880 | 8.849924e-04 | 2571.920600 |
2497 | 998800 | 4994 | 2133.01740 | -154.30520 | 17789.2956 | -3136.7632 | -2999.1820 | 8143.76800 | 7694.591800 | -2558.05720 | 0.000905 | 0.000879 | 8.856225e-04 | 2572.948200 |
2498 | 999200 | 4996 | 2104.37720 | 3917.94820 | 17789.2956 | -3131.6026 | -2995.8686 | -4305.08920 | 1851.551560 | -2325.52240 | 0.000906 | 0.000879 | 8.854430e-04 | 2573.974000 |
2499 | 999600 | 4998 | 2114.60280 | -3419.91340 | 17789.2956 | -3141.3600 | -3004.9664 | -3060.68180 | -142.958434 | 1462.89470 | 0.000905 | 0.000879 | 8.847412e-04 | 2575.006400 |
2500 | 1000000 | 5000 | 1957.24718 | -4201.52280 | 17789.2956 | -3141.0764 | -3014.8324 | -505.61330 | 1229.747960 | 1879.30786 | 0.000905 | 0.000879 | 8.845744e-04 | 2576.046600 |
2501 rows Ă— 14 columns
We can apply a function to the DataFrame. The default is the columns (axis=0). Either way, we get a new DataFrame.
def minmax(roworcolumn):
return np.min(roworcolumn), np.max(roworcolumn)
df.apply(minmax)
Step | v_mytime | Temp | Press | Volume | PotEng | TotEng | v_pxy | v_pxz | v_pyz | v_v11 | v_v22 | v_v33 | CPU | |
---|---|---|---|---|---|---|---|---|---|---|---|---|---|---|
0 | 0 | 0 | 883.05875 | -6247.6768 | 8894.6478 | -1576.8745 | -1513.6955 | -6747.6227 | -7547.8097 | -7509.4468 | 0.000013 | -0.000564 | 1.295104e-07 | 0.0000 |
1 | 500000 | 2500 | 1154.17900 | 6673.7634 | 8894.6478 | -1559.1555 | -1488.9586 | 7428.1767 | 6523.4548 | 6229.5043 | 0.001115 | 0.000531 | 9.109558e-04 | 1288.0233 |
Here we analyze across the rows.
df.apply(minmax, axis=1)
0 (-1566.6216, 8894.6478)
1 (-2203.3768, 8894.6478)
2 (-1564.3755, 8894.6478)
3 (-1566.4325, 8894.6478)
4 (-1569.3172, 8894.6478)
...
2496 (-2265.505, 499200.0)
2497 (-1568.3816, 499400.0)
2498 (-2152.5446, 499600.0)
2499 (-1709.9567, 499800.0)
2500 (-2100.7614, 500000.0)
Length: 2501, dtype: object
Summary¶
Pandas is a multipurpose data science tool. In many ways it is like a numpy array, and in many ways it is different. In some ways it is like a dictionary.
The similarities include the ability to do some indexing and slicing. This is only a partial similarity though.
The differences include integrated plotting.